Algorithmic trading has revolutionized financial markets, leveraging artificial intelligence (AI) and machine learning (ML) to execute trades at speeds and efficiencies previously unimaginable. These technologies have allowed for the creation of sophisticated algorithms that can process vast amounts of data, adapt to market changes, and optimize trading strategies.
This article explores the evolution of algorithmic trading, the role of AI and ML in enhancing trading capabilities, and their future potential in reshaping financial markets.
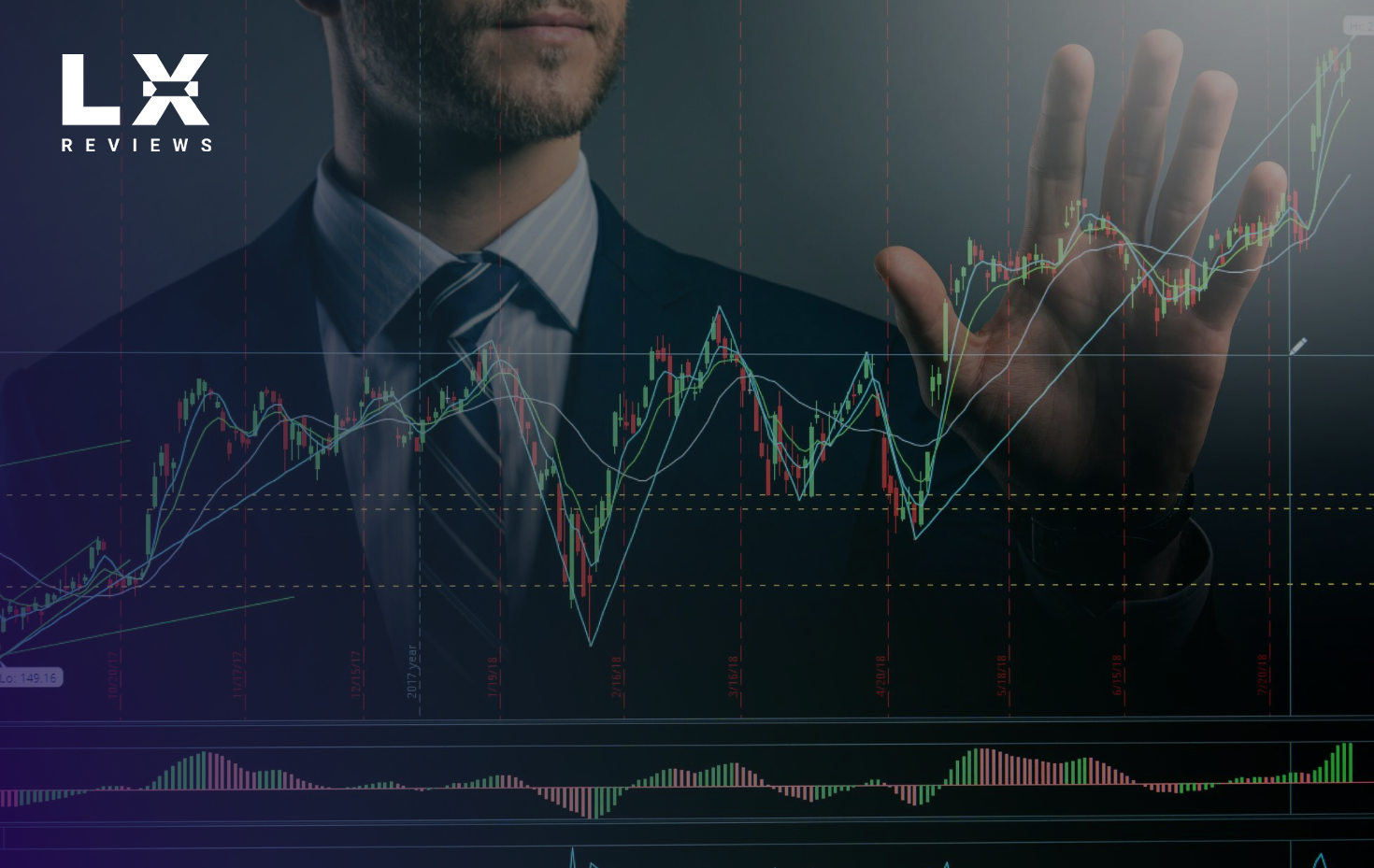
The Origins of Algorithmic Trading:
Algorithmic trading emerged with the rise of electronic trading systems in the 1970s. Before computers became integral to finance, trades were manually executed on the trading floors by brokers. The advent of electronic exchanges allowed automation of key processes such as order matching and trade execution. Early algorithms were simple, relying on basic rules like price limits and volume constraints.
Over time, as market data grew in complexity, trading strategies became more intricate, leading to the birth of high-frequency trading (HFT), where thousands of orders could be executed in fractions of a second.
AI and Machine Learning in Algorithmic Trading
1. Application of Machine Learning Algorithms in Trading
Machine learning, a subset of AI, focuses on building systems that can learn from data and improve over time. In trading, machine learning algorithms analyze vast volumes of historical and real-time market data to identify patterns and correlations that may not be immediately apparent to human traders.
- Supervised Learning: Machine learning models are trained using labeled data to predict future price movements or market behavior based on past data. These models are useful for trend analysis, correlation detection, and anomaly identification.
- Unsupervised Learning: Algorithms process unstructured data to discover hidden trends in the market. As the system learns, it becomes more adaptable to changing market conditions and can adjust its strategies accordingly.
2. Sentiment Analysis using AI
AI-powered sentiment analysis tools are transforming how traders interpret market conditions. By analyzing news articles, social media posts, and other forms of unstructured data, AI algorithms can gauge market sentiment in real time. This sentiment—whether positive, negative, or neutral—can influence market movements, and traders can use this data to anticipate price changes.
For example, a negative sentiment regarding a company’s earnings report might prompt an algorithm to short its stock in anticipation of a price drop.
3. Reinforcement Learning in Trading
Reinforcement learning (RL) is another AI-driven method gaining traction in algorithmic trading. RL involves algorithms learning from their environment through trial and error, optimizing their strategies based on feedback from their actions. This approach enables algorithms to improve continuously, making them highly effective in dynamic and unpredictable markets.
RL systems can fine-tune trading strategies by learning from past trades, identifying profitable approaches, and discarding ineffective ones. This adaptability allows RL algorithms to respond efficiently to ever-changing market conditions.
Advantages of AI and Machine Learning in Algorithmic Trading
The integration of AI and machine learning into algorithmic trading has brought numerous advantages to traders, institutions, and financial markets as a whole:
1. Speed and Efficiency
AI algorithms can process massive amounts of data in real time, allowing for ultra-fast decision-making and trade execution. In high-frequency trading (HFT), where success often depends on speed, AI can place trades in milliseconds, capitalizing on even the smallest price movements.
2. Improved Accuracy and Precision
AI-powered systems eliminate human errors that may arise from emotional biases or fatigue. These algorithms rely solely on data inputs and pre-defined rules, allowing them to make precise and accurate trades without the influence of external factors. Over time, AI algorithms learn from fresh data, improving their accuracy and performance.
3. Enhanced Risk Management
AI systems can identify and assess potential risks by analyzing both historical and current market data. Machine learning algorithms can predict market downturns or volatility spikes, allowing traders to manage risk proactively. AI-driven systems can automatically adjust positions or exit trades before potential losses accumulate.
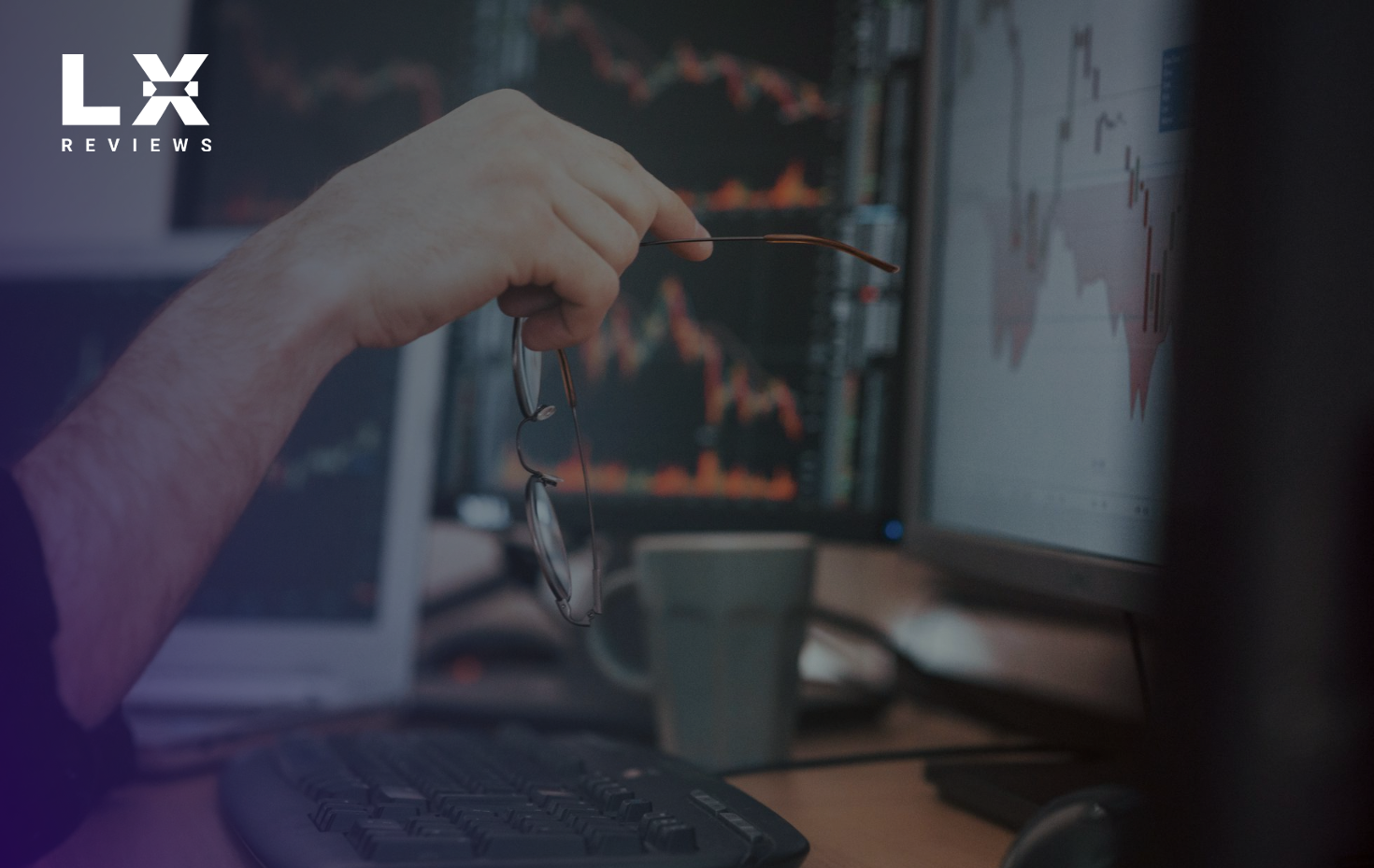
Challenges and Risks of AI and Machine Learning in Trading
Despite the benefits, AI and machine learning come with several challenges and risks that traders must navigate:
1. Data Quality and Overfitting
The effectiveness of machine learning models is heavily dependent on the quality of data. If the data used to train algorithms is incomplete or flawed, the predictions made by the models may be inaccurate. Overfitting, where a model is too closely tailored to historical data, is another issue that can reduce the model’s ability to adapt to new market conditions.
Ensuring clean, high-quality data and using techniques like cross-validation can help mitigate these risks.
2. System Failures and Black Swan Events
AI systems are vulnerable to technical failures or market shocks. In instances where an algorithm malfunctions, or a black swan event (e.g., an unexpected market crash) occurs, significant losses can result. The 2010 Flash Crash, where automated trading algorithms contributed to a rapid and extreme market downturn, is a reminder of the risks associated with algorithmic trading.
To address this, traders and institutions must implement safeguards, monitor algorithms in real time, and develop contingency plans for unexpected events.
3. Ethical Concerns and Market Manipulation
The rise of AI-powered trading systems has raised concerns about market manipulation. Algorithms capable of executing trades in milliseconds can be used to manipulate market prices or engage in practices like front-running. Regulators are increasingly focused on ensuring that AI-driven trading operates ethically and does not undermine market integrity.
The Future of AI and Machine Learning in Algorithmic Trading
The future of algorithmic trading is intrinsically tied to the continued evolution of AI and machine learning. As these technologies advance, algorithms will become even more sophisticated, capable of handling increasingly complex market scenarios. The advent of quantum computing may further enhance AI’s ability to process data and make real-time decisions at an even faster pace.
Additionally, we may see greater collaboration between human traders and AI systems. Hybrid approaches, where human traders work alongside AI to make strategic decisions, could lead to more efficient and profitable trading strategies.
Conclusion
AI and machine learning have significantly transformed the landscape of algorithmic trading. By improving speed, accuracy, and risk management, these technologies have enabled traders to make better decisions, optimize strategies, and execute trades with unprecedented efficiency.
However, challenges related to data quality, system failures, and ethical concerns remain. Moving forward, AI and machine learning will continue to evolve, reshaping financial markets and offering new opportunities for profitability and efficiency.